Historically speaking, even before AI and Large Language Models (LLMs) became cool, recommendation systems were one of the earliest use cases for AI.
The rise of LLMs has made building a recommendation system 100 times easier. What used to take months can now be done in a few days with some prompt engineering.
What makes things even easier is the framework laid out in this paper: RecPrompt: A Prompt Tuning Framework for News Recommendation Using Large Language Models.
Even if you aren’t building a recommendation system on top of LLMs, the principles in the RecPrompt framework can be applied to many AI use-cases.
What is RecPrompt
RecPrompt is a prompt engineering framework designed to enhance news article recommendations.
RecPrompt has three main components: A Prompt Optimizer, a Recommender, and a Monitor.

The Recommender generates news recommendations, which, along with the initial prompt, are fed into the Prompt Optimizer.
The Optimizer refines the prompt based on the example recommendations provided, enhancing alignment with user preferences based on previous recommendations. This creates a feedback loop that leads to responses that are more in line with the user’s click history.
The Monitor measures and records the effectiveness of the newly generated prompt against specific performance metrics like Mean Reciprocal Rank (MRR) and others.
Below are some of the exact prompts used.
System Message Prompt
Initial Prompt Template
We also put together a template you can use directly in PromptHub. It is based off the template above, with some structural enhancements.
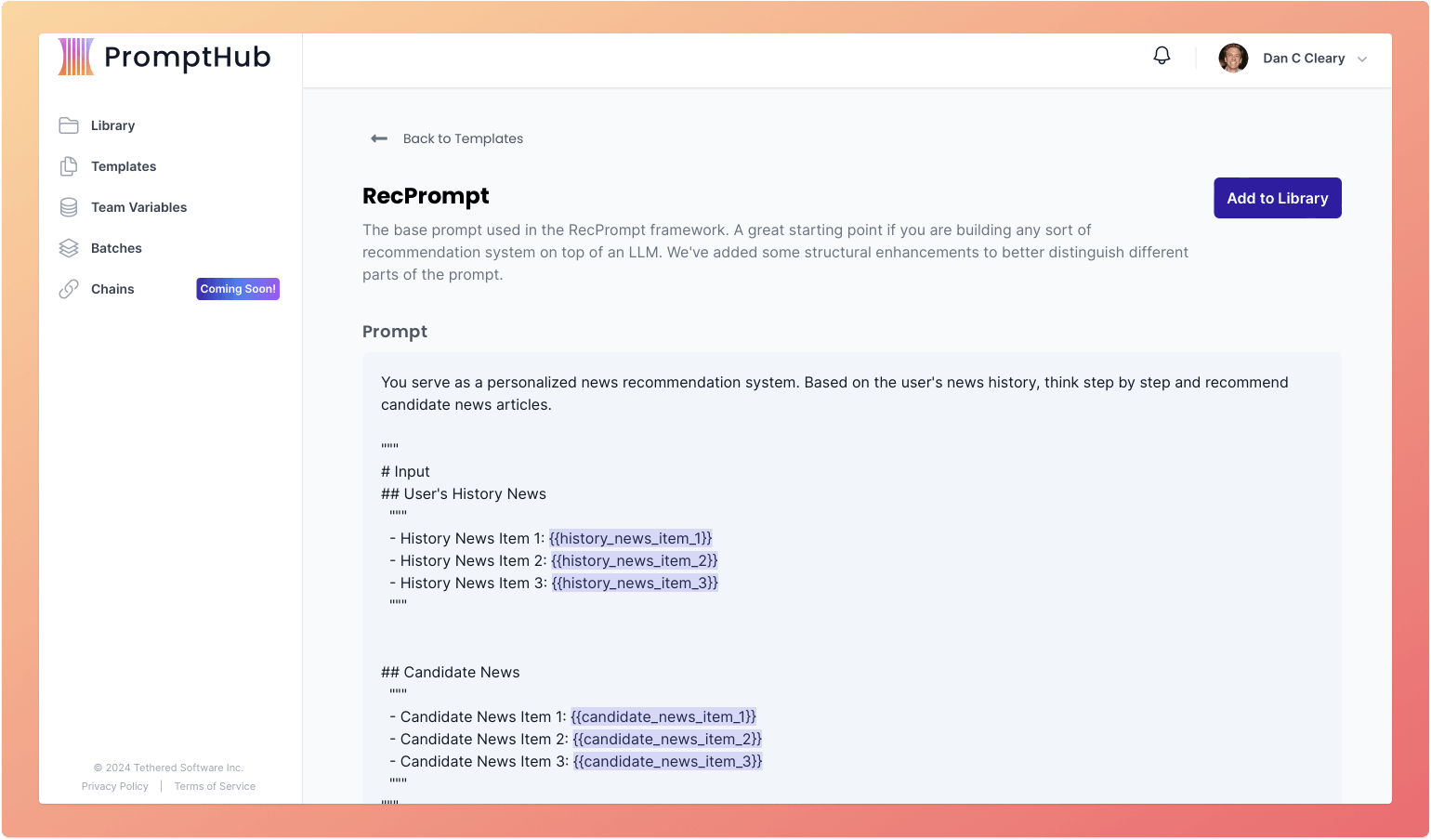
Prompt Engineering Techniques used in RecPrompt
A very cool aspect of the RecPrompt paper is their testing of two methods for the prompt optimizer: manually tuning the prompt and letting an LLM update the prompt.
We’ve talked a few times about using LLMs to optimize prompts, (Using LLMs to Optimize Your Prompts, How to Optimize Long Prompts).
We believe LLMs can be great prompt optimizers, but we’ve seen the best results when combining humans and LLMs.
Manual prompt engineering method
RecPrompt starts with an initial prompt template. This template is then manually updated during the optimization process. This involves tweaking things like the instructions and descriptions to improve the LLM's understanding and recommendation performance.
LLM-based prompt engineering method
This method automates the prompt iterating process using another LLM.
The Prompt Optimizer refines the initial prompt template by integrating four components into a single input for the LLM:
- The system message
- The current candidate prompt template
- A set of samples from the recommender
- An observation instruction (meta-prompt) which guides the LLM to adjust the prompt template to better align with the desired improvements and recommendation objectives.
The output from the optimizer is the enhanced prompt template.
The refined prompt is submitted to the recommender LLM to make news recommendations.
Experiment set up
The researchers put RecPrompt to the test across various datasets to evaluate its effectiveness.
Datasets
- Microsoft News Dataset (MIND): Collection of news articles
Evaluations
- RecPrompt’s performance was evaluated across a few metrics: AUC, MRR, nDCG@5, and nDCG@10
Implementation Details
- Models Used: GPT-3.5 and GPT-4
Baselines
RecPrompt was compared against a few news recommendation methods and deep neural models.
News recommendation methods
- Random: Randomly recommends candidate news to the user
- MostPop: Recommends news based on the randomly selected views, aggregating the total number of views across the dataset
- TopicPop: Suggests popular news articles based on the user’s browsing history.
Deep neural models
Below are the deep neural models tailored for news recommendations. It’s not very important to understand the intricacies, but here is a little info on each.
- LSTUR: Integrates an attention-based Convolutional Neural Network (CNN) for learning news representations with a GRU network
- DKN: Employs a Knowledge-aware CNN for news representation and a candidate-focused attention network for recommendations.
- NAML: Uses dual CNNs to encode news titles and bodies, learning representations from text, category, and subcategory, with an attention network for user representations.
- NPA: Features a personalized attention-based CNN for news representation, coupled with a personalized attention network for user modeling.
- NRMS: Applies multi-head self-attention mechanisms for news representation and user modeling.
Experiment Results
Alright, let’s take a look at the results.
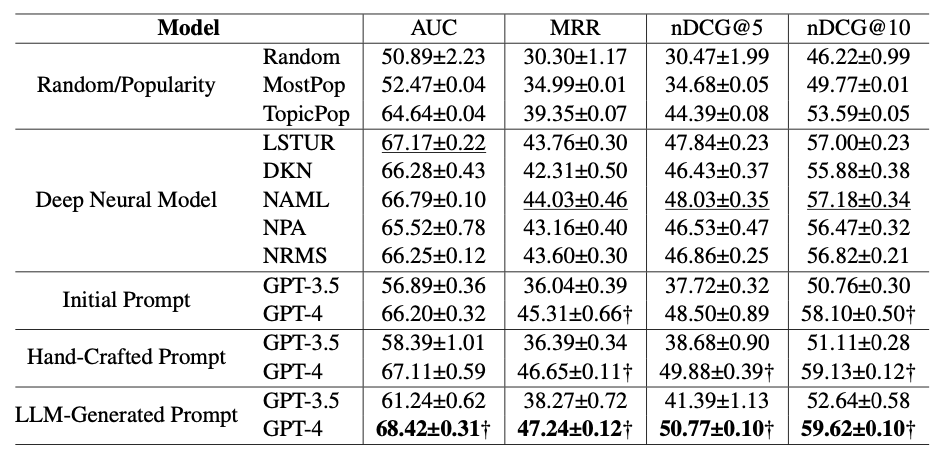
Takeaways
- TopicPop is the top performer in the first group of methods, probably due to the fact leverages users's browsing history to provide more tailored recommendations
- All the deep neural model methods outperform TopicPop
- AML, on average, outperforms all other methods in its group
- Initial Prompt with GPT-3.5 performs worse than TopicPop and all the deep neural models
- Initial Prompt with GPT-4 outperforms most deep neural models
- There is a clear pattern when it comes to effectiveness: LLM-Generated Prompt > Hand-Crafted Prompt > Initial Prompt.
- GPT-4 is a better prompt optimizer compared to GPT-3.5
- The only LLM based recommendation method that beats all of the deep neural models is LLM-Generated Prompt using GPT-4
Here's my favorite part
Let's dive deeper on the incremental improvements of the three prompt based methods. We’ll focus on just one of the evaluation sets (MRR, because I’m a SaaS founder), although the trends are similar across all the sets.
GPT-3.5 performance on MRR
Initial Prompt: 36.04
Hand-Crafted Prompt: 36.39 .97% increase compared to Initial Prompt
LLM-Generated Prompt: 38.27 6.19% increase compared to Initial Prompt
GPT-4 performance on MRR
Initial Prompt: 45.31
Hand-Crafted Prompt: 46.65 2.96% increase compared to Initial Prompt
LLM-Generated Prompt: 47.24 4.26% increase compared to Initial Prompt
If you need to recall what the Initial Prompt is, I’ve copied it below for reference:
It’s just a single prompt. Compared to the other methods it is easier to implement. Recreating the larger prompt engineering framework in full is neither extremely challenging nor trivial.
The percentage differences in performances can be seen as large or small in relation to the work needed to implement the framework. It really depends on your use case. If Google can make Google Search .00001% faster, that’s a big deal. But if your use case isn't as sensitive to smaller percentage gains, or if you just need to get something out quickly, I would advise to use GPT-4 and leverage the basic prompt template above.
Wrapping up
RecPrompt can teach us a few things about prompt engineering. The automated framework is a good use case of using LLMs to optimize prompts, in a way that is structured and has a recurring feedback loop.
Sometimes the most interesting takeaways from studies like these are pretty simple. The absolute easiest way to get better results is to use GPT-4. Depending on your use case, implementing a framework like RecPrompt and be the boost that makes your product more compelling than your competitors, or it may not be neccessary as a starting point. Either way, now you have the tools and knowledge to decide!
