Retrieval-Augmented Generation, also known as RAG, is a way to provide specific context to a Large Language Model when sending your prompt. I recently put out a video on RAG and in my research I found most RAG content out there to be either:
- Overly technical
- Really long
So for those that prefer text over video, and concise and not super technical overviews, this one is for you! We’ll dive into what RAG is, how it works and a few examples with some beautiful flow charts. Plus we’ll also go over how to set up a very simple RAG pipeline with just a few lines of (open-source) code.
Understanding RAG
Retrieval Augmented Generation, or RAG, is a method to optimize the output from an LLM by supplementing its static knowledge with external, up-to-date information.
LLMs are powerful but, once trained, they don’t automatically update with new information. RAG fixes that by retrieving relevant data (think news articles, or company 10-Ks) and feeding it to the model alongside your prompt.
How RAG Works: A step-by-step overview
The graphic looks more complex than it actually is. Let’s break it down.
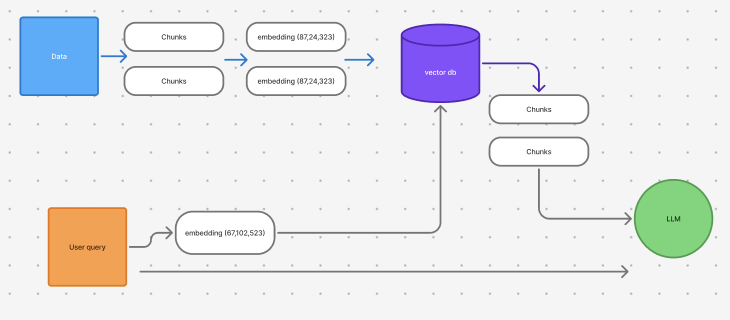
1. Data processing and embedding generation
- Data Source (blue square): Your process begins with raw documents—this could be PDFs, web pages, or other text data.
- Chunking: Large documents are split into smaller, manageable chunks. This makes it easier for the system to handle and retrieve specific pieces of information.
There are many different ways to handle chunking data, it can be tricky. For example, if we added some documentation as a data source and half of a code example was in chunk 1 and the other half in chunk 2, that will cause some issues. - Embedding Conversion: Each chunk is transformed into a numerical vector (an embedding) that captures its semantic meaning. What’s an embedding? We’ll touch on this more later, but you can also learn more about it here: A Beginner's Guide on Embeddings and Their Impact on Prompts
2. Vector database role
Once converted, these embeddings are stored in a vector database—a specialized storage system (purple cylinder). This database lets the system quickly search for and retrieve the chunks that are most relevant to a given query.
3. User query transformation & retrieval
- Query transformation: When a user asks a question, that query is also converted into an embedding.

- Retrieval: The system then searches the vector database for chunks whose embeddings are similar to the query’s embedding—meaning they are close to each other in the vector space. These relevant chunks then provide context for the LLM.
For reference, here is a visualization of the word “dog” in a vector space, showing how semantically related words cluster around it.
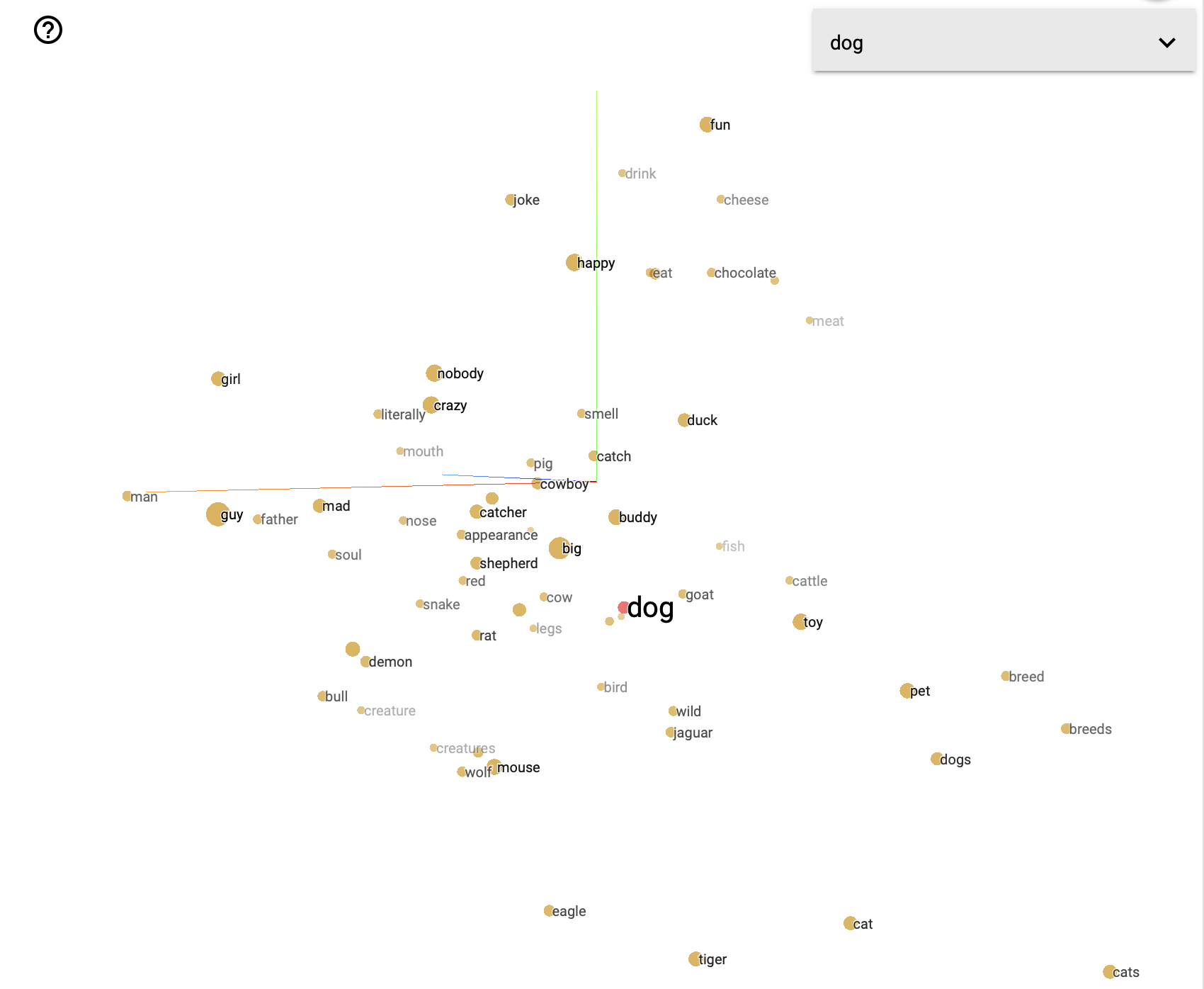
Here are the closest points in the vector space, showing the words that appear most similar to “dog.”

4. Send it all to the LLM
The retrieved chunks—converted back to text, along with the original query—are then fed into the LLM. This enriched context enables the model to generate responses that are both more accurate and comprehensive.
Simple RAG workflow
Put more succinctly, here is how RAG works.
- Data → Chunking → Embeddings: Raw data is transformed into embeddings, which are stored in the vector database.
- Query Processing: A user query is converted into an embedding, which is then used to retrieve the most relevant data chunks by comparing the similarity between embeddings.
- LLM Integration: The LLM receives both the query and the retrieved context to generate a final output.
Retreival Augmented Generation example: Up-to-date financial services assistant
Let’s run through a quick example.
Imagine you built a chatbot or an app that is a financial assistant. This assistant will need up to date information on publicly traded companies. These sources of information could be 10-Qs (quarterly) and 10-Ks (annual). Here’s how you could use RAG to make this efficient.
Flow breakdown
- Processing the filings: All filings are chunked, converted into embeddings, and stored in a vector database. We consistently add new filings as they are released.
- Targeted Retrieval: When the user asks a question related to recent financial information about a company, the system retrieves only the relevant chunks from the filings.
- Enhanced Response: The LLM then provides an answer based on the latest information, that it wouldn’t have access to otherwise (knowledge cutoff).
Setting up a simple RAG pipeline in 5 minutes
Part of what drew me to RAG now was that I wanted to stand up a quick pipeline to test it out. I was able to do so in just a few lines of code and had it running in less than 20 minutes.
Here is a link to the repository that has the code, and I walk through it in my video here. But here is a general overview of the pipeline.
- Data Loading: Load your documents using a reader (using LlamaIndex).
- Chunking & Embedding: Break down the docs into chunks and convert to embeddings.
- Storage: Save these embeddings in a vector database for fast retrieval.
- Query Handling: Convert user queries into embeddings and search the vector database.
- Response Generation: Pass both the query and retrieved data to the LLM to generate a response.
Conclusion
RAG is just one method you can use to optimize LLM performance. I personally am not very bullish on RAG. As context windows get larger, and models become faster and cheaper, I think you’ll see more use of Cache-Augmented Generation (CAG), otherwise know as “shove it in the context window”. But that analysis is for another time and another blog post (soon)!
