Context windows are getting longer and longer. A few weeks ago, Google announced that Gemini 2.5 Pro would support a 2-million-token context window. Just a week later, Meta announced that their new Llama 4 Scout model would support a whopping 10-million-token context window. We’re clearly trending towards larger and larger context windows.
As context windows expand, what does this mean for Retrieval-Augmented Generation (RAG)? If we can support millions of tokens in the context window, what does that mean for RAG? Can we simply load all the context and use Cache-Augmented Generation (CAG) to cache the context upfront?
The Mechanics of RAG vs. CAG
Let’s quickly level set on RAG and CAG. Also, if you’re looking for a deeper dive on RAG, check out our guide here: Retrieval Augmented Generation for Beginners.
Retrieval-Augmented Generation (RAG)
In a traditional RAG setup, you begin with a collection of documents/files. These files are then broken down into smaller, manageable chunks. Here’s the typical process:
- Data chunking and embedding: The documents are segmented into smaller chunks. Each chunk is transformed into a vector using an embedding model. These embeddings capture the semantic essence of the text.
- Vector database storage: The embeddings are stored in a vector database.
- Dynamic retrieval at query time: When a user sends a prompt, the query is also transformed into a vector. The vector database is then searched for the most semantically relevant passages. These passages are retrieved in real time.
- Prompt formation: The retrieved snippets are concatenated with the user’s query and sent to the LLM.
Pros and cons of RAG:
- Pros:
- Reduces the number of tokens processed in each query, which lowers costs
- Easy to dynamically and frequently update the content in the vector database to handle new data. Especially useful when the information changes frequently
- Its modularity makes it easy to test individual pieces
- Cons:
- Introduces many extra steps into the whole system, which has a number of downsides (more failures points, can increase latency, complexity of setup and maintenance)
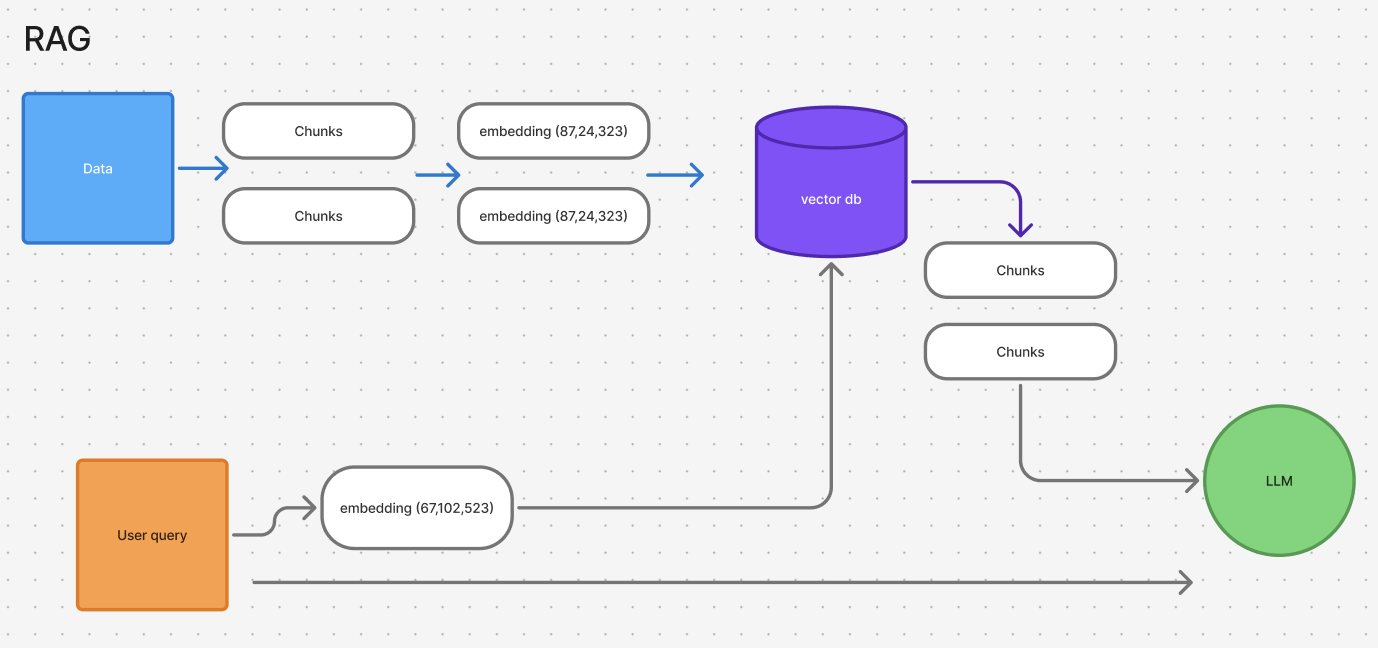
Cache-Augmented Generation (CAG)
CAG takes a different approach by leveraging large context windows and caching mechanisms to supply information to the LLM.
- Preloading and pre-computation: All documents are loaded into the model’s extended context window. For open-source models, you can precompute and store these documents in the model’s key-value (KV) cache, effectively ‘freezing’ its internal state.
On the other hand, when using a proprietary model via an API, you typically don't have direct access to manipulate the KV cache. You'll need to use the provider’s built-in caching mechanisms (see more details on prompt caching here).
- Inference without retrieval: Now when a user sends a prompt, it’s essentially appended to the preloaded context. This allows the model to process the entire cached context along with the new prompt.

Pros and cons of CAG:
- Pros:
- Faster inference times (no retrieval needed)
- Potentially more robust answers since the whole context is shared. This is dependent on how well the model can handle the number of tokens in the context window.
- Simplified system design with no need for a separate retrieval component.
- Cons:
- Limited by the context window of whatever model you are using
- Can be more expensive depending on how often the context updates
- It’s less dynamic. If the underlying data changes often, you may need to reload the context and recompute the cache, which can be expensive.
Performance Analysis: CAG vs. RAG
Alright now we’ll dive into some data the experiments ran in this paper: Don’t Do RAG:When Cache-Augmented Generation is All You Need for Knowledge Tasks
Experiment set up
The experiments evaluated RAG and CAG approaches on several datasets by gradually increasing the total length of the reference texts. Here are the details:
- Baseline RAG Implementation: Using the LlamaIndex framework, two retrieval strategies were used:
- Sparse Retrieval (BM25): Relies on keyword matching.
- Dense Retrieval (OpenAI Indexes): Uses embedding-based semantic search.These methods dynamically fetch and concatenate document chunks at query time.
- CAG Implementation: The entire set of documents is preloaded into the model’s key-value (KV) cache.
Experiment results
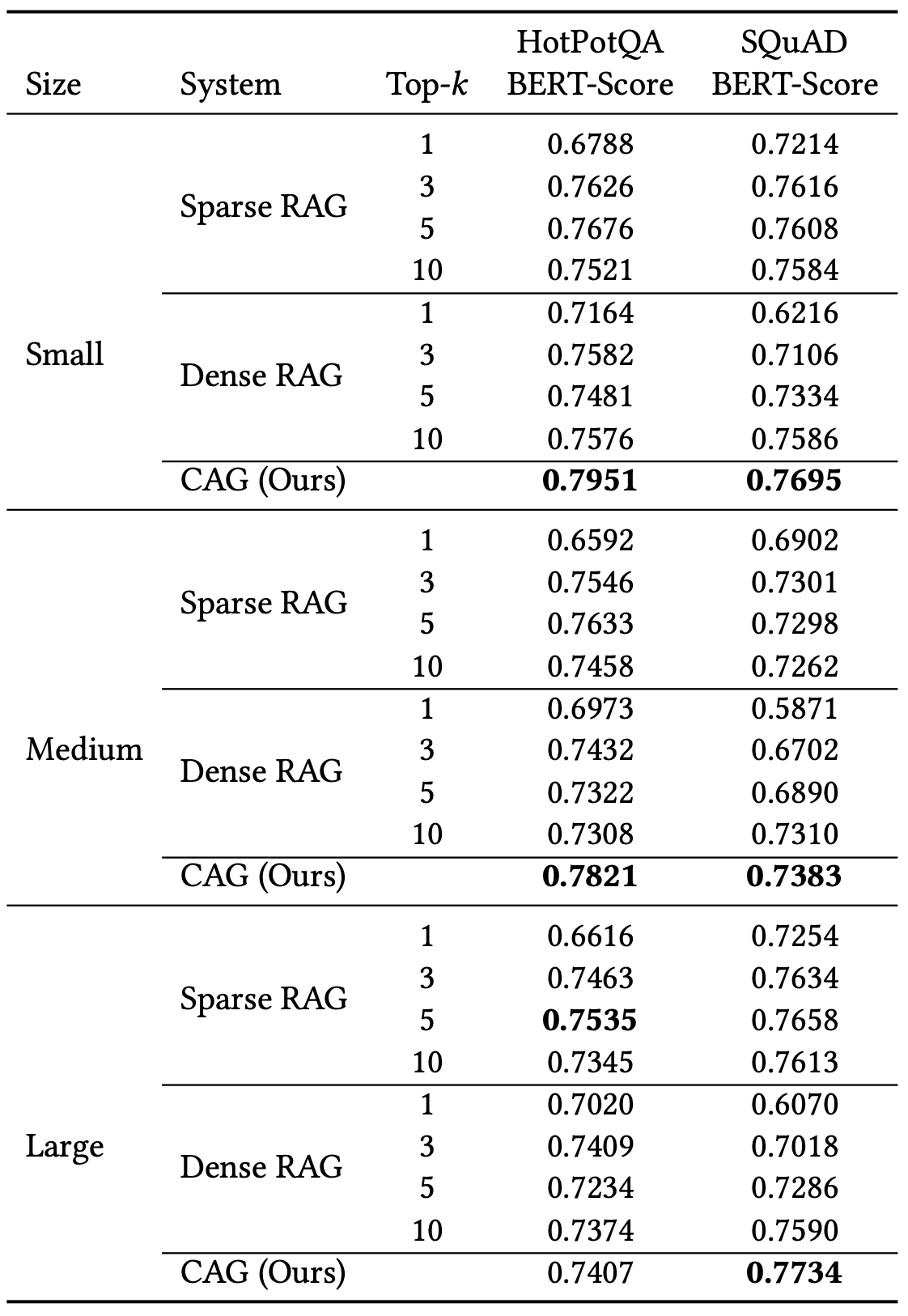
- Overall CAG outperforms RAG in almost every experiment
- As the size of the reference text increases, the performance gap between CAG and RAG narrows slightly
- The gap is pretty small in some cases, but the difference between RAG and CAG isn’t just about performance, it’s also about implementation, cost, and latency

- CAG greatly reduces generation time by eliminating retrieval overhead

One more graph, from a different paper Retrieval Augmented Generation or Long-Context LLMs? A Comprehensive Study and Hybrid Approach.
The experiment setup is very similar. A few RAG methods versus preloading the entire reference corpus via CAG, called "Long Context (LC)" in this paper.
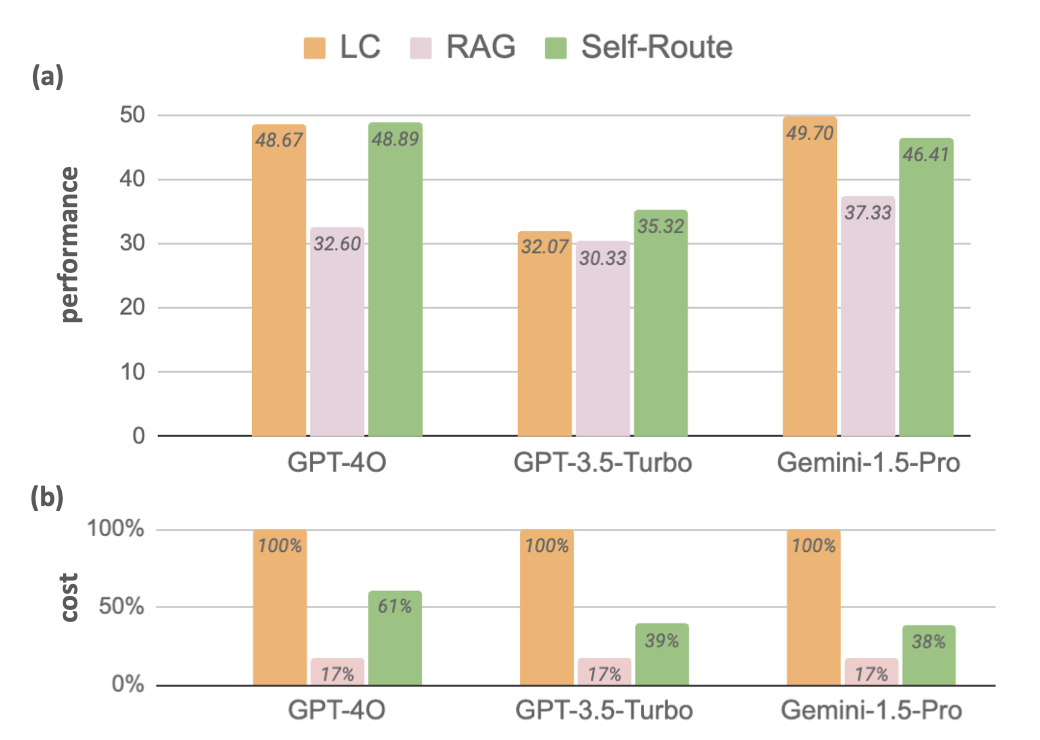
- Again, CAG (LC) consistently outperforms RAG
- CAG is more expensive than RAG when it comes to that first request when caching the reference corpus. Subsequent requests will be less expensive than RAG
Common Failure Modes for RAG:
The study also mentioned four common reasons why RAG fails on certain queries.
- Multi-step Reasoning (A): Queries that require chaining multiple facts.
- General Queries (B): Broad or vague questions, making it hard for the retriever to pinpoint relevant chunks.
- Long, Complex Queries (C): Deeply nested or multi-part questions that the retriever struggles to interpret.
- Implicit Queries (D): Questions requiring a holistic understanding beyond explicit mentions (e.g., inferring causes in narratives).
Conclusion
So which method should you use? It’s dependent on your use case, but I think CAG makes for a good starting point because of its simplicity. A question we didn’t touch too much on though is, how effective are the models at actually handling hundreds of thousands—or even millions—of tokens? Subscribe to our newsletter or visit our blog next week to learn more!